本文已被:浏览 936次 下载 996次 |
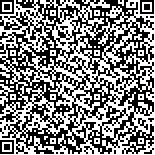 码上扫一扫! |
脑电非线性动力学和多频段功能连接网络特征随运动性疲劳累积的变化研究 |
张陈,冉令华,呼慧敏,张欣,周子健,徐红旗,史冀鹏 |
(东北师范大学 运动能力测评与提升研究中心,长春 130024;中国标准化研究院 人类工效学实验室,北京 100191 ;国家市场监督管理总局重点实验室 (人因与工效学),北京 100820;吉林大学 仪器科学与电气工程学院,长春 130061) |
|
摘要: |
目的:探究脑电非线性动力学和多频段功能连接网络特征随运动性疲劳累积的变化。方法:11名受试者采用50%1RM 进行重复下肢蹬伸至力竭的肌肉耐力测试,同步采集用于疲劳阶段划分的 BorgCR-10量表和脑电信号。按照不同疲劳阶段对脑电进行处理。①提取香农熵、对数能量熵、Renyi熵、Tsallis熵、θ波绝对能量、α波绝对能量、β波绝对能量和 (θ+α)/β指标;②在 θ (4~8Hz)、α (8~12Hz)和β (12~30Hz)频段,两两导联计算获得PLV 和WPLI 值,通过阈值构建脑功能连接网络,进一步利用平均度、网络效率、特征向量中心性和聚类系数进行拓扑结构分析;③对比上述指标在不同疲劳阶段的差异。结果:香农熵在 Fp1和 Fp2的轻度与重度疲劳间存在统计学差异 (p<0.05);对数能量熵除了在 C3和 P3导联外,均在不同疲劳程度之间表现出统计学差异 (p<0.05);θ波绝对能量、β波绝对能量和 (θ+α)/β分别在不同导联的不同疲劳程度之间表现出统计学差异 (p<0.05);β频段的脑功能网络连接逐渐增强;由 WPLI值构建的脑功能连接网络在α和β频段的复杂网络指标在不同疲劳程度表现出统计学差异和显著相关 (p< 0.05)。结论:单一频段的绝对能量仅在重度疲劳发生显著变化,而非线性动力学指标和复杂网络指标则随疲劳加深表现出阶梯式变化,体现了后者对于疲劳变化监测的敏感性,可作为疲劳评估与预警的有效生物指标。 |
关键词: 脑电 非线性动力学 功能连接 疲劳 拓扑特性 |
DOI: |
投稿时间:2024-12-23 |
基金项目:国家重点研发计划课题 “形态数字标准人模型构建技术研究” (2023YFF0615902);国家市场监管重点实验室 (人因与工效学 ) 开放基金 “上肢工作姿态心理物理学负荷评估模型研究 ”(2023SYSKF02002);国家市场监管总局科技计划项目 (2023MK192);中央基本科研业务费项目(522023Y-10381) |
|
Research on the Evolution of Nonlinear Dynamics and Multi-bandFunctional Connectivity Network Features with the Accumulation ofExercise-Induced Fatigue |
ZHANG Chen,RAN Linghua,HU Huimin,ZHANG Xin,ZHOU Zijian,XU Hongqi,SHI Jipeng |
(Research Centre for Assessment and Enhancement of Athletic Ability, Northeast Normal University, Changchun 130024 , China;Ergonomics Laboratory, China National Institute of Standardization, Beijing 100191 , China ;SAMR Key Laboratory of Human Factors and Ergonomics, Beijing 100820 , China;College of Instrumentation and Electrical Engineering, Jilin University, Changchun 130061 , China) |
Abstract: |
Objectives: This study aims to explore the changes of EEG nonlinear dynamics and multibandfunctional connectivity network features with the accumulation of exercise-induced fatigue.Methods: Eleven participants underwent lower limb muscle endurance tests to exhaustion, utilizing50% of their one repetition maximum (1RM). Simultaneous recordings of Borg CR-10 scale ratingsand electroencephalographic (EEG) signals were collected for fatigue stage segmentation. EEG wasprocessed according to different fatigue stages. ①Shannon entropy, logarithmic energy entropy, Renyientropy, Tsallis entropy, θ wave absolute power, alpha wave absolute power, beta wave absolutepower, and the (θ + α) / β index were extracted; ②in the θ (4~8 Hz), α (8~12 Hz), and β (12-30 Hz) frequency bands, the Phase Locking Value (PLV) and Weighted Phase Lag Index (WPLI)values were calculated by two-lead calculation, and the brain functional connection network was constructedby threshold, followed by topological structure analysis incorporating mean degree, networkefficiency, eigenvector centrality and clustering coefficient; ③this paper made a comparison of theaforementioned metrics across different fatigue stages. Results: Statistically significant differences inShannon entropy were observed between mild and severe fatigue in channels Fp1 and Fp2 (p<0.05).Logarithmic energy, except for channels C3 and P3, exhibited statistically significant differencesacross various fatigue levels (p<0.05). θ wave absolute power, α wave absolute power, and (θ +α) / β demonstrated statistically significant differences across different fatigue levels in distinct channels(p<0.05). The strength of brain functional network connections in the β frequency band increasedgradually. Brain functional connectivity networks constructed from WPLI values showed statisticallysignificant differences and significant correlations across different fatigue levels in the α and βfrequency bands (p<0.05). Conclusion: While absolute power in a single frequency band significantlychanged only during severe fatigue, nonlinear dynamics and complex network metrics displayedstepwise alterations with increasing fatigue, highlighting their sensitivity for monitoring fatigue changesand their potential as effective biomarkers for fatigue assessment and early warning. |
Key words: electroencephalography (EEG) nonlinear dynamics functional connectivity fatigue topologicalfeatures |